Good Enough Isn’t an Option
Reliability and performance are mission-critical. In aerospace, robotics, and advanced manufacturing, precision isn’t negotiable. Today’s systems demand insight far beyond traditional monitoring. Yet, the path to true observability has been bogged down by unnecessary complexity and rising costs. The challenge isn’t just understanding the past—it’s cutting through the noise. Hardware observability provides the solution.
Hardware observability isn’t a buzzword—it’s a tactical edge. It dives into the inner workings of machines, catching problems before they escalate into catastrophic failures. This approach transforms systems from ‘good enough’ to exceptional.
This guide outlines the fundamentals of hardware observability: its core components, its advantages, and the challenges to be aware of when scaling up. Whether tuning a rover or safeguarding a spacecraft, observability is the key to dominating any domain.
Monitoring is Dead
Monitoring is reactive. It only reveals what’s been pre-programmed to track, missing the bigger picture. For simple systems, it suffices. For complex, interconnected hardware systems, it falls short.
- Monitoring: Like a check engine light, it signals an issue but provides no details. It can track known metrics, but it leaves engineers blind to root causes. Troubleshooting becomes a guessing game.
- Observability: Dynamic and proactive, observability pulls in all system-generated data—telemetry, logs, traces—allowing new questions to be asked in real time. It catches problems before they escalate.
Monitoring tells you what went wrong after the fact. Observability shows you why it happened and how to stop it from happening again.
For complex systems like autonomous vehicles, spacecraft, or industrial machines, this shift isn’t optional—it’s the difference between reacting to problems and preventing them from ever occurring.
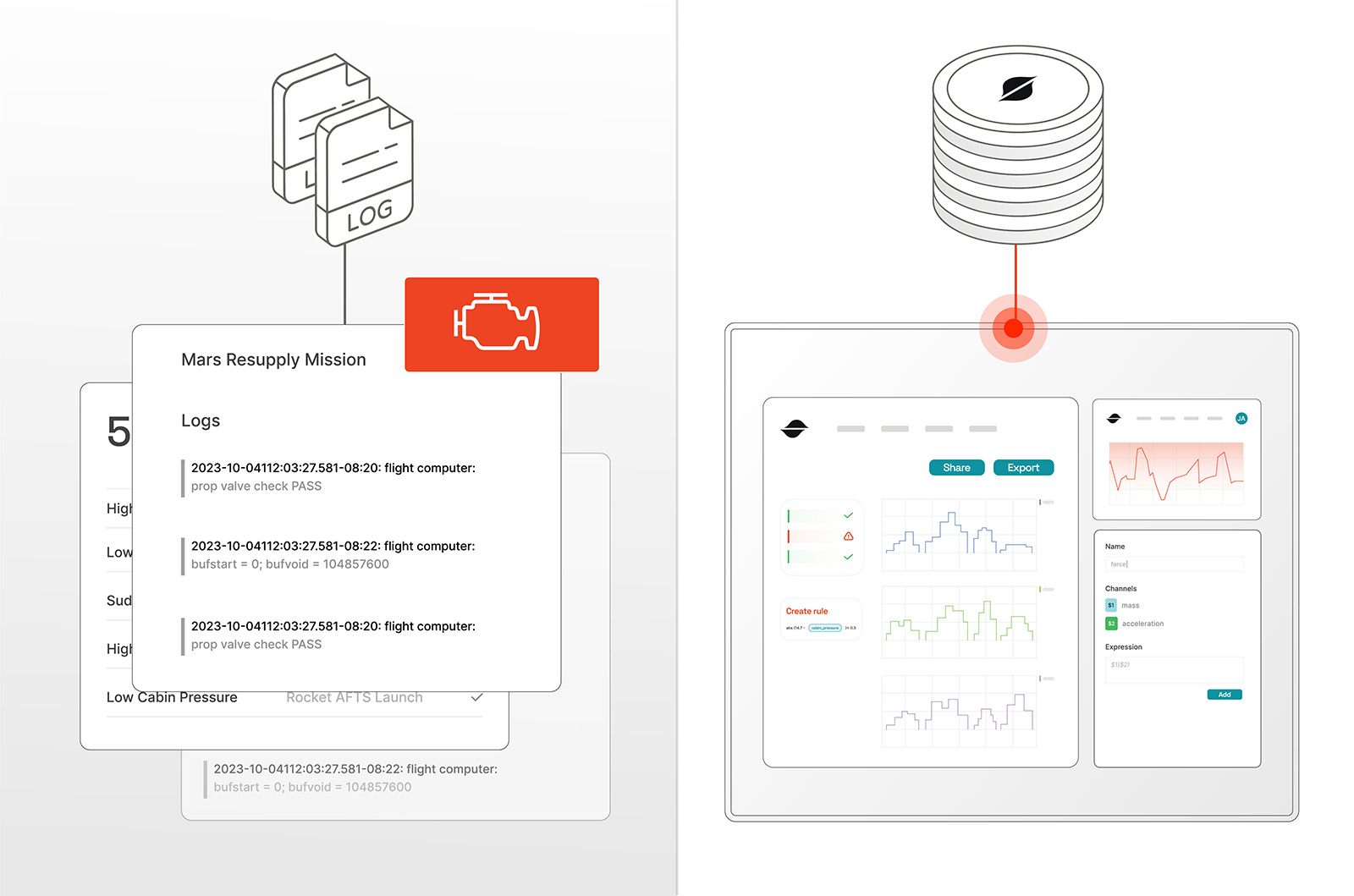
Observability allows immediate diagnosis and resolution of problems, bypassing the need for endless dashboard reviews.
Cracking Open the Black Box
Hardware observability is about analyzing a system’s outputs—telemetry, logs, real-time data—to understand its behavior. Collecting data is not enough. Without actionable insights, it’s impossible to surface unknowns or prevent issues from escalating. Observability allows immediate diagnosis and resolution of problems, bypassing the need for endless dashboard reviews.
The Building Blocks of Observability
At the heart of hardware observability are three core pillars: telemetry data, metrics, and traces. Each of these plays a critical role in giving you the visibility needed to monitor, troubleshoot, and optimize your systems—but with them comes complexity.
- Telemetry Data: Raw data from machines—sensor readings, performance metrics, logs, and real-time telemetry. Telemetry offers granular insight into hardware behavior. As data scales, without the right tools, the noise can be overwhelming.
- Metrics: Numerical representations of system performance over time. Metrics show high-level health indicators like CPU load or temperature thresholds. In complex systems, metrics lead to high-dimensional data problems known as cardinality explosion, making management and analysis increasingly difficult.
- Traces: Traces map the path of data or signals through a machine or network. They are vital for understanding how commands and data propagate, especially in distributed systems. Traces introduce complexity, as sifting through massive datasets becomes the norm.
Traditional monitoring systems, designed for IT and software, fail in hardware environments.
Hardware Observability: A Game-Changer
Observability isn’t a rebranded version of monitoring. Traditional monitoring systems, designed for IT and software, fail in hardware environments. Observability offers proactive insights, revealing the unexpected, not just the obvious. In hardware, failure can lead to missed launch windows—or worse. Observability ensures early detection and smooth, uninterrupted operations.
Unlocking the True Potential of Modern Machines
Observability, when managed properly, transforms operations:
Precise Anomaly Detection: Rules-based anomaly detection ensures that operational irregularities are identified and addressed before they escalate. By codifying nominal behavior, the system flags off-nominal conditions with minimal false positives, reducing unnecessary alerts.
Enhanced Root Cause Analysis: Observability takes guesswork out of problem-solving, pinpointing the root cause of issues quickly. Teams can investigate problems with precision, dramatically reducing mean time to resolution (MTTR).
Data-Driven Collaboration: With unified data sharing across all teams, observability enhances collaboration between engineering, operations, and leadership. A consistent, real-time foundation eliminates silos and enables faster, more informed decisions.
Streamlined Reporting & Certification: Observability ensures compliance and operational readiness through automated reporting and certification tools. Engineers can generate detailed reports with a click, saving time and maintaining system reliability without the manual overhead.
Challenges
Modern machines bring significant observability challenges. Without addressing these head-on, the true potential of observability will never be realized:
- Data Volume and Complexity: The sheer scale of telemetry data can quickly spiral out of control. In fact, 63% of survey respondents identified data management challenges as a ‘some’ or ‘major’ consequence. Without advanced aggregation, high-performance storage like Sift DB, and smart pipelines, you’ll end up buried under a mountain of noise instead of actionable insights.
- Integration with Existing Systems: Adding new observability tools to existing infrastructure can be a nightmare and lead to operational inefficiencies. 77% of respondents reported inefficiencies from siloed tools and fragmented data. Seamless integration through flexible APIs and careful planning is crucial to avoiding data silos.
- Skill and Knowledge Gap: Not every team has the specialized skills to fully utilize observability. 62% of respondents acknowledged gaps in their ability to manage data complexity and anomaly detection. Training and upskilling are non-negotiable if you want to extract the full value from your observability investment.
- Balancing Real-time Insights with Cost: Relying on patchwork systems or open-source tools might seem like a quick win, but it leads to inefficiencies and rising costs as you scale. 66% of respondents expressed concerns over escalating storage costs and scalability issues. An integrated platform like Sift solves these problems, delivering reliable, real-time insights without the long-term financial drain.
- Human Factors and Cognitive Load: Engineers aren’t machines, and when they’re overwhelmed with data, critical signals get missed. 62% of respondents reported challenges with anomaly detection and false alerts. Systems should be designed to reduce cognitive load, giving engineers the context they need without overloading them.
- Long-term Scalability and Flexibility: What works today won’t necessarily work tomorrow. 66% of respondents raised concerns about scalability. Observability strategies must be adaptable to growing data, new technologies, and evolving architectures.
For more insights on challenges, view the 2024 Aerospace Observability Report
Real-World Example: The Helium Incident
A team we worked with was able to keep their spacecraft schedule on track despite a major anomaly by utilizing their observability system. During standard fueling operations, their system detected a flight computer malfunction. Upon further investigation, they realized helium molecules leaking from the tanks slipped their way into the clock of the flight computer, causing the quartz crystals to oscillate irregularly—a scenario no one had anticipated.
Traditional monitoring systems, relying on predefined dashboards, would have missed this critical interaction. You will only be monitoring it if you know what to look for. However, the observability platform surfaced this unexpected behavior, allowing engineers to trace the anomaly to its source and resolve it before it could jeopardize the mission.
This incident underscores the vital role of observability in modern hardware development. In industries where the stakes are high, observability platforms like Sift are not just useful—they’re essential.
Traditional monitoring systems, relying on predefined dashboards, would have missed this critical interaction.
Take Control
If you’re ready to take control and uncover the unknowns, Sift is here to help. Our platform gives you the tools to surface hidden issues in real time, enabling smarter data analysis and proactive troubleshooting. It’s time to move beyond monitoring and embrace a more powerful, insightful way to build modern machines.